Beyond One-Size-Fits-All: The Future of AI Specialization
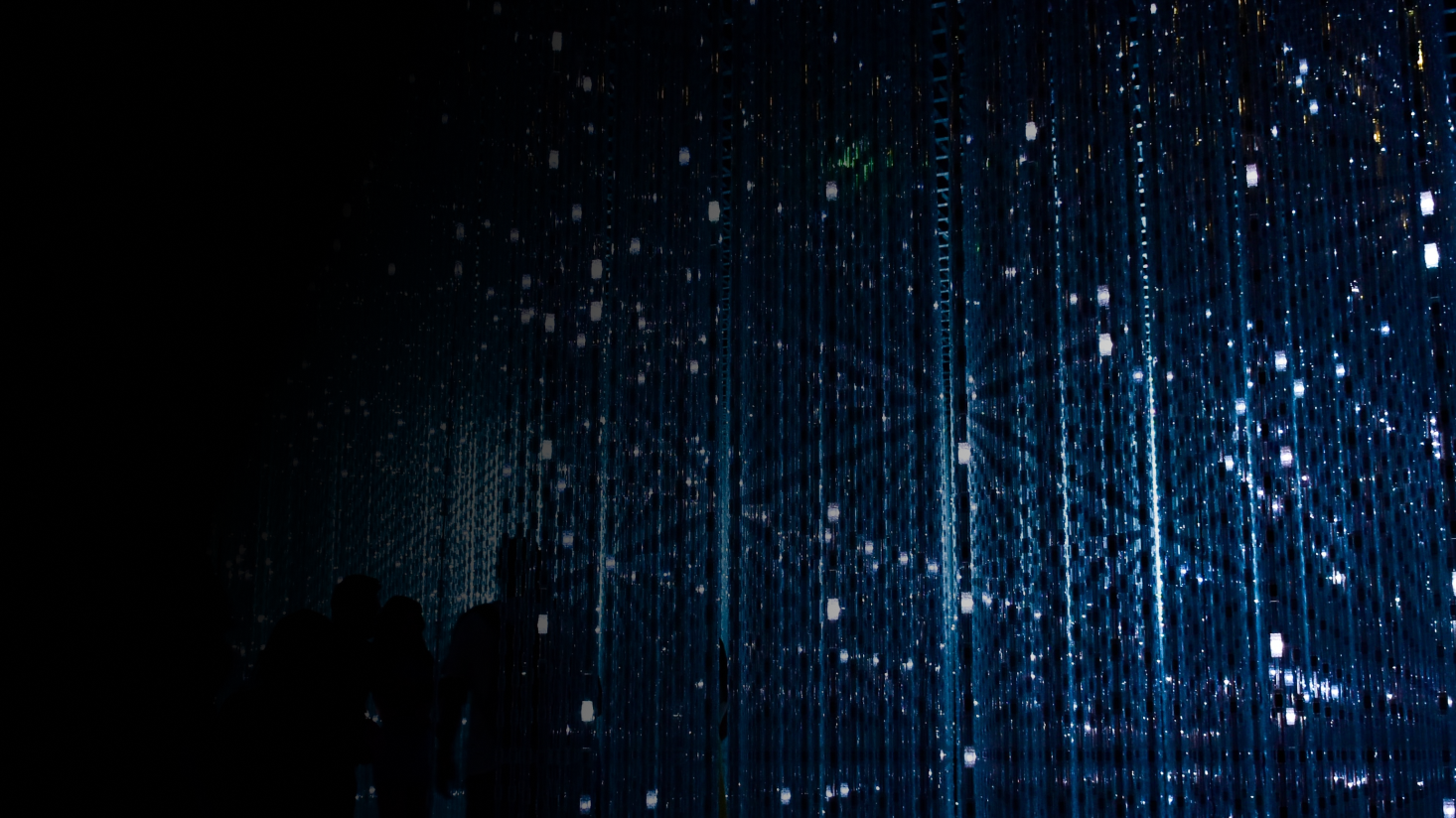
General AI tools democratize artificial intelligence but create a surprising barrier: they're so powerful and versatile that using them effectively requires becoming an expert. This expertise paradox sits at the heart of today's AI revolution, where the most accessible tools often demand the most specialized knowledge.
The parallel with programming is striking. If you can write code, you can make a computer do virtually anything. That's an incredibly powerful interface which democratizes computing. But to use that one-size-fits-all interface of code, you must become an expert programmer. That's incredibly complex, which is why most people don't build software. Instead, they use software that someone else has customized, where that more complex interface has been transformed into something usable.
The Hidden Expertise Barrier
AI experts know how to write incredibly complex prompts. They know how to string together prompts to produce far more complex outputs over time. The average person simply doesn't know how to do this. They don't know all the different best practices for each step in producing incredible work using AI. So they're actually at an enormous disadvantage.
Because of where we are in the market cycle, all the tools out there are lower-level, one-size-fits-all. But ultimately, that's not where the industry is heading.
At Nomad Data, we've helped many companies use artificial intelligence to perform specific tasks. The people ultimately doing those jobs who would most benefit from automation are typically not very technical. Even when they have some technical know how, they aren’t necessarily experts at artificial intelligence.
Our goal at Nomad Data has been to create a highly customizable engine that can be quickly tuned to perform specific tasks. By teaching the system the complex steps and hiding AI prompts in the platform, you don't have to teach people to become AI experts. This makes the tools simpler to use because they've already been tuned to do something specific.
From Generic Prompts to Specialized Solutions
Consider our due diligence tool for mergers and acquisitions. Banks and private equity funds often deal with thousands of documents around an acquisition. With a one-size-fits-all tool, they might give a generic prompt: "Look through all these documents and put together the summary I need."
Then they'll sit down and try to think through all the different ways to describe that summary after their first prompt returns something generic and unhelpful. It takes a long time, if ever, to truly articulate it in a way that produces what they want. And the underlying system might not even be capable of doing that.
What we've learned at Nomad is that a better approach is for an expert to sit down with them, understand exactly what they're looking to extract from that array of documents, and quickly launch what we call a "preset." This is essentially a customized AI application on our platform that leverages our technology to solve their company specific problem. Users don't have to tell it anything special; they just point it at the deal room and say "go."
Anyone can get up to speed on this very quickly. Before such customized solutions were available, you had to teach every person to become an AI prompt engineer. That's just not scalable, especially as the technology changes and models improve. Users would constantly need to update their skills to take advantage of these tools.
The Coming Explosion of Specialized Vendors
Just like in software, AI specialization will lead to an explosion in vendors. That's where you create competitive barriers as a company. You specialize and build features that a specific industry or use case cares about. We're just in the very early innings of this industry developing, which is why you haven't seen that happen yet.
The switch we're looking for is vendors developing very specific features that appeal to a much narrower set of people. We'll also reach the point where it becomes clear to customers that they cannot build these solutions themselves.
In the very early days of a technology like this, everything seems easy to build because we're at the lowest layer of abstraction. There isn’t years of features built up around the underlying technology. As we transition up those layers of abstraction, people will build more complex systems around the underlying AI models. Eventually, it becomes very challenging for companies to compete from scratch. Customers stop competing with vendors, and you see more of a traditional software ecosystem emerge.
The Cognitive Load Difference
The cognitive difference between using general AI and specialized AI is similar to writing code versus using an application. If I have a problem and a background in computer science, I can spend 20 minutes writing some pretty advanced code, which is basically a prompt. I'm creating a set of instructions to solve that problem.
Alternatively, someone else could write that same code, release it as a production application where someone clicks a button, and the same thing happens. Writing that code requires an incredible amount of specialized skill, which doesn't make sense for everybody to have.
The same is true with AI. It doesn't make sense for every worker who wants to benefit from AI to become an absolute expert in it. All of this complexity, all of this prompting, is eventually going to be hidden behind UIs and buttons.
It won't be one UI where you tell it to do something and it can do everything. That's not feasible given the fragmentation of knowledge and context and the differences between roles, which are in many cases not even well documented. It would be very hard to train a model to do everything.
In some far-off future state, that might be possible. But it's not a technology problem. It's a data problem. What everyone does, how every process works, is simply not well-recorded in a way that model companies can access and train models to handle.
Capturing Specialized Knowledge
The best method for developing specialized AI is to sit down and walk hand-in-hand with the client through the process being optimized. This is done by someone who is an expert in AI and is learning the customer's problem.
They then create presets in Nomad Data’s Doc Chat which are trained not only from an AI perspective but also on the workflow and different steps involved. Over time, you work with the client to see where the system deviates from what they would have done, and you capture that in future releases.
Managing Bias in Specialized AI
With specialized AI, you know the exact problem the AI is being used to solve. This makes it easier to understand how various biases introduced into the system could affect outcomes in these specific cases.
It's much harder when a solution is being used for an unknown number of different tasks to imagine the biases in all of them. Specialized solutions are easier to make transparent because, again, you know exactly what they're being used to solve.
Overcoming Negative Experiences
Currently, almost everyone is adopting one-size-fits-all tools, which gives customers some exposure to AI. But this can turn negative when users try to implement these general tools for specialized tasks and don't succeed. That leaves a bias in customers' minds about what AI is capable of doing well.
Just today, during a client onboarding at Nomad Data, one of the champions on the account had to point out to colleagues that they had used a one-size-fits-all tool unsuccessfully. They encouraged others not to let that experience bias what they thought was possible with AI.
We usually explain that one-size-fits-all tools are mostly consumer-grade products. They cost very little per person, so you can't expect them to do everything. Nomad Data's approach, customizing AI to a particular problem, is more of an enterprise-grade tool. As a result, it's also more expensive.
For a system to perform these more complex tasks, customers understand that it should cost more. It doesn't make sense that you could pay $10 a month, or even use a free product, that replaces thousands of man-hours of work. That's something people can internalize once confronted with the reasoning.
The Future AI Landscape
I think we'll end up with more and more specialized AI tools, but I don't even think we'll call them "specialized AI tools." They'll just be tools that happen to employ AI to perform tasks.
In some cases, it may be existing vendors you already work with that come up the learning curve on AI and make it easier to use. But it's also very possible that this will be a highly disruptive cycle in which many small companies displace larger ones because of their AI-first mentality.
Preparing for the Specialized AI Future
So far, the most important thing we've seen is a willingness by customers to explore how this technology can transform their work. Organizations that are open to and accepting of the idea that the world is about to change dramatically are typically the ones to start embracing this change more quickly, often to their advantage.
Teaching basic artificial intelligence skill sets is helpful: what is a large language model? What is generative artificial intelligence? What is prompting? What are examples of good prompts versus bad prompts?
But more important than technical skills is the organizational mindset that recognizes we're at the beginning of a fundamental shift. The specialized AI revolution won't just change how we use technology. It will change who can use it, democratizing capabilities that today remain locked behind expertise barriers.
The future won't belong to those who can write the most sophisticated prompts, but to those who can identify the specific problems AI can solve in their domain. As specialized AI tools proliferate, the competitive advantage will shift from technical AI expertise to deep domain knowledge paired with an understanding of where AI can be most effectively applied.
We're moving from a world where AI is a tool wielded by experts to one where AI expertise is embedded in the tools themselves. This transition will make artificial intelligence truly accessible to everyone, not just in theory but in practice.