Agricultural Buildings Data
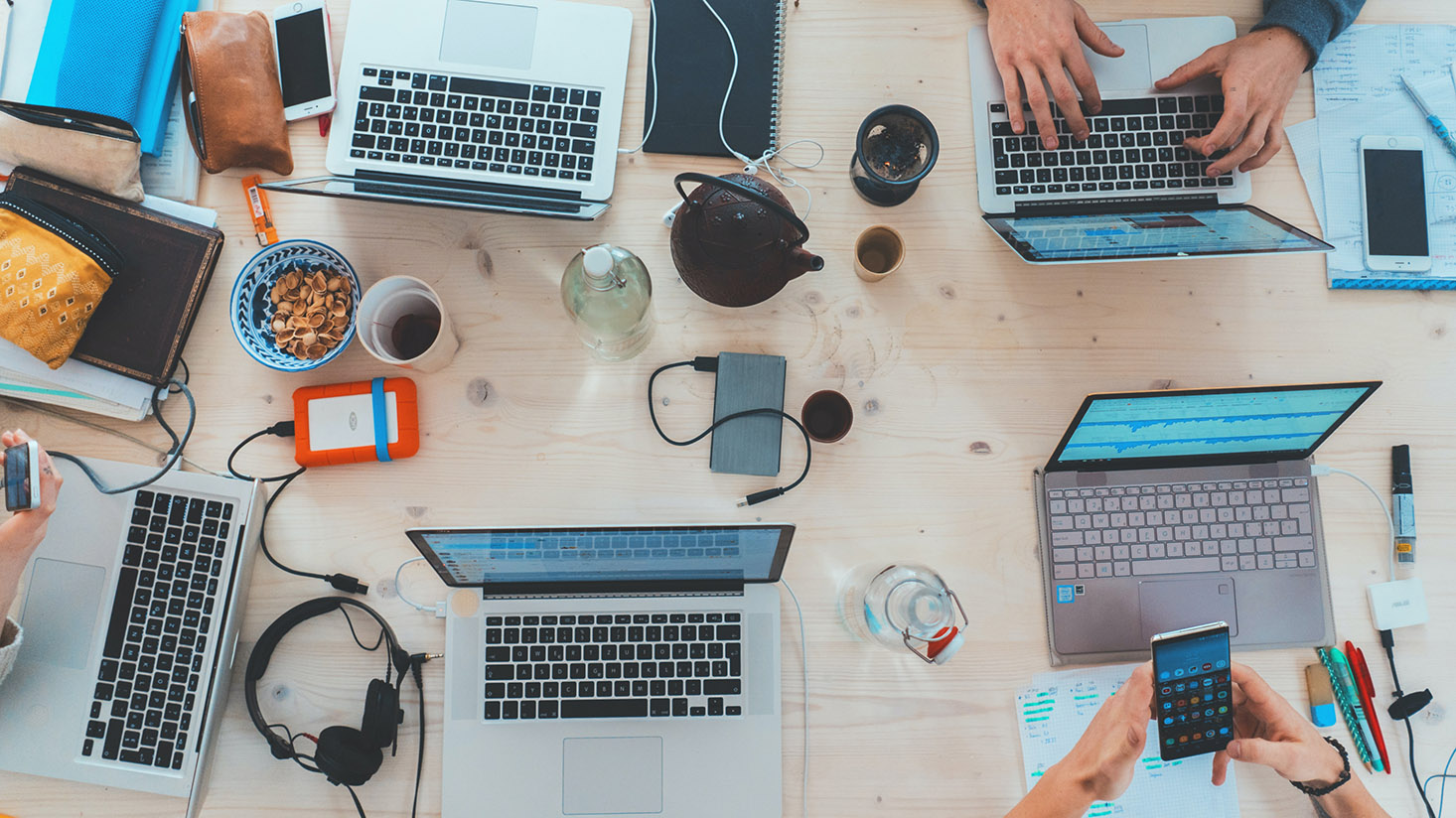
Introduction
Understanding the landscape of commercial farming in the U.S. has historically been a challenge fraught with reliance on outdated methods and scarce data. Before the digital age, insights into agricultural operations, including the total number of buildings on commercial farms, were gathered through manual surveys and anecdotal evidence. This approach not only lacked precision but also timeliness, leaving stakeholders in the dark about real-time changes and trends in the sector.
The advent of sensors, the internet, and connected devices has revolutionized data collection, making it easier to gather detailed information about agricultural operations. Previously, the absence of data meant relying on generalized agricultural reports or conducting time-consuming physical assessments of farms. The proliferation of software and databases has now enabled the storage and analysis of every event happening on a farm, from crop yields to the specifics of farm infrastructure.
The importance of data in understanding agricultural operations cannot be overstated. In the past, weeks or even months could pass before changes in agricultural practices or infrastructure were understood. Now, data allows for real-time insights, enabling stakeholders to make informed decisions swiftly. This shift has not only improved operational efficiency but also enhanced strategic planning and resource allocation in the agricultural sector.
However, navigating the vast ocean of available data to find relevant insights about agricultural buildings and operations can still be daunting. This article aims to shed light on specific categories of datasets that can provide valuable insights into the U.S. commercial farming landscape, focusing on the total number of buildings on site.
From geolocation and location data to diversified and real estate data, various data types offer unique perspectives on agricultural operations. By understanding the history, technology advances, and application of these data types, stakeholders can gain a comprehensive view of agricultural infrastructure and its implications for the sector.
The transformation from antiquated data collection methods to modern, data-driven insights marks a significant evolution in agricultural understanding. This article will explore how different data types contribute to this understanding, providing stakeholders with the tools they need to navigate the complexities of commercial farming in the U.S.
Geolocation Data
Geolocation data has become an invaluable resource in the agricultural sector, offering precise information about the location and characteristics of farm buildings. Historically, understanding the spatial distribution of agricultural infrastructure required extensive fieldwork and manual mapping. The introduction of geospatial technologies and GIS (Geographic Information Systems) has revolutionized this process, enabling the collection and analysis of detailed spatial data.
Examples of geolocation data relevant to agricultural buildings include parcel data, GIS spatial boundaries, building footprints, and ownership and address information. This data can provide insights into the number of buildings on each parcel, their placement, and other critical characteristics such as size, shape, and land use code.
Industries and roles that benefit from geolocation data in agriculture include farm managers, real estate developers, policy makers, and agricultural researchers. The technology advances that facilitated the emergence of geolocation data include satellite imagery, drones, and advanced computing capabilities for processing large datasets.
The amount of geolocation data available for agricultural purposes is accelerating, thanks to continuous updates and the integration of new data sources. This data can be used to:
- Assess farm infrastructure: Understand the spatial layout and number of buildings on commercial farms.
- Land use planning: Aid in decision-making for land development and conservation efforts.
- Resource allocation: Optimize the distribution of resources based on the spatial characteristics of farms.
- Market analysis: Provide insights into agricultural real estate trends and opportunities.
Geolocation data offers a comprehensive view of agricultural buildings, enabling stakeholders to make informed decisions about farm management, development, and investment.
Location Data
Location data provides another layer of insight into agricultural operations, combining address data, parcel boundaries, building footprint polygons, and assessor data. This data type builds on the foundation laid by geolocation data, offering a more granular view of agricultural infrastructure.
Historically, location data was limited to basic mapping and surveying techniques. The advent of digital mapping and advanced data collection methods has expanded the scope and accuracy of location data, making it a critical tool for understanding agricultural buildings.
Examples of location data applications in agriculture include zoning analysis, structure counting, and land use assessment. This data is particularly useful for identifying agricultural parcels and the buildings associated with them, providing a detailed inventory of farm infrastructure.
Roles and industries that benefit from location data in agriculture range from urban planners and environmental consultants to agricultural economists and farm owners. The technology advances that have enabled the proliferation of location data include high-resolution satellite imagery, aerial photography, and sophisticated data analytics platforms.
The use of location data in agriculture is growing, driven by the need for precise information about farm structures and land use. This data can be used to:
- Identify agricultural parcels: Determine land zoned for agricultural use and associated buildings.
- Infrastructure analysis: Analyze the type and distribution of buildings on agricultural parcels.
- Regulatory compliance: Ensure adherence to zoning and land use regulations.
- Investment decisions: Inform investment strategies based on detailed infrastructure data.
Location data enhances the understanding of agricultural buildings, offering valuable insights for planning, management, and investment purposes.
Diversified Data
Diversified data encompasses a wide range of information relevant to agricultural operations, including farm size, grain storage capacity, and building details. This category of data provides a holistic view of agricultural infrastructure, combining elements of geolocation, location, and operational data.
Before the availability of diversified data, stakeholders relied on fragmented information sources to piece together a comprehensive picture of agricultural operations. The integration of diverse data sources into unified platforms has streamlined this process, offering a more complete and accessible view of farm infrastructure.
Examples of diversified data in agriculture include farm operation details, storage capacities, and building information. This data is crucial for understanding the operational capacity and infrastructure of commercial farms.
Industries and roles that benefit from diversified data in agriculture include agribusiness companies, supply chain managers, agricultural lenders, and policy analysts. The technology advances that have facilitated the collection and analysis of diversified data include IoT (Internet of Things) devices, cloud computing, and big data analytics.
The volume of diversified data in agriculture is increasing, driven by the demand for comprehensive insights into farm operations and infrastructure. This data can be used to:
- Operational planning: Optimize farm operations based on detailed infrastructure and capacity data.
- Supply chain management: Enhance supply chain efficiency through better understanding of storage and distribution infrastructure.
- Risk assessment: Evaluate the risks associated with agricultural infrastructure and operations.
- Strategic decision-making: Inform strategic decisions with a holistic view of agricultural operations.
Diversified data offers a comprehensive perspective on agricultural buildings and operations, enabling stakeholders to make informed decisions about farm management and strategy.
Real Estate Data
Real estate data provides insights into the ownership, valuation, and characteristics of agricultural properties, including buildings. This data type is essential for understanding the market dynamics and investment opportunities in the agricultural sector.
Historically, real estate data was gathered through public records and manual surveys, limiting the scope and timeliness of available information. The digital transformation of the real estate industry has made detailed property data more accessible, offering valuable insights into agricultural buildings and land.
Examples of real estate data relevant to agriculture include property ownership records, valuation data, and building characteristics. This data is crucial for assessing the value and potential of agricultural properties.
Roles and industries that benefit from real estate data in agriculture include investors, developers, appraisers, and agricultural economists. The technology advances that have enabled the widespread availability of real estate data include online property databases, digital mapping, and advanced analytics tools.
The use of real estate data in agriculture is expanding, driven by the need for accurate and timely information about property values and characteristics. This data can be used to:
- Property valuation: Assess the value of agricultural properties based on detailed building and land data.
- Market analysis: Analyze trends and opportunities in the agricultural real estate market.
- Investment strategy: Inform investment decisions with comprehensive property data.
- Development planning: Plan agricultural development projects based on property characteristics and market dynamics.
Real estate data enhances the understanding of agricultural buildings and properties, offering valuable insights for investment, development, and market analysis purposes.
Conclusion
The importance of data in understanding agricultural operations and infrastructure cannot be overstated. The transition from antiquated data collection methods to modern, data-driven insights has transformed the agricultural sector, enabling stakeholders to make informed decisions in real time.
Access to diverse types of data, including geolocation, location, diversified, and real estate data, provides a comprehensive view of agricultural buildings and operations. These data types offer unique perspectives and insights, contributing to a deeper understanding of the agricultural landscape.
As organizations become more data-driven, the ability to discover and leverage relevant data will be critical to success in the agricultural sector. The potential for data monetization also presents new opportunities for stakeholders to capitalize on valuable insights generated from decades of agricultural operations.
The future of agricultural data is promising, with emerging technologies and data types poised to offer even more detailed insights into agricultural operations. The integration of AI and machine learning technologies has the potential to unlock the value hidden in historical data and modern government filings, further enhancing the understanding of agricultural infrastructure and operations.
In conclusion, data plays a pivotal role in the agricultural sector, offering the insights needed to navigate the complexities of commercial farming. By embracing data-driven approaches, stakeholders can enhance operational efficiency, strategic planning, and investment decisions, ultimately contributing to the growth and sustainability of the agricultural sector.
Appendix
Industries and roles that could benefit from agricultural data include investors, consultants, insurance companies, market researchers, and agricultural economists. The challenges faced by these industries, such as understanding market dynamics, assessing risk, and optimizing operations, can be addressed through the strategic use of data.
Data has transformed the agricultural sector by providing detailed insights into farm infrastructure, operations, and market trends. This transformation has enabled stakeholders to make informed decisions, improve efficiency, and identify new opportunities.
The future of agricultural data is bright, with AI and machine learning technologies offering the potential to unlock the value hidden in decades-old documents and modern government filings. These technologies can provide deeper insights into agricultural operations, enhancing decision-making and strategic planning.
As the agricultural sector continues to evolve, the importance of data-driven approaches will only increase. Stakeholders who leverage data effectively will be well-positioned to navigate the challenges and opportunities of the agricultural market, driving innovation and growth in the sector.