Churn Prediction Enhancement Data
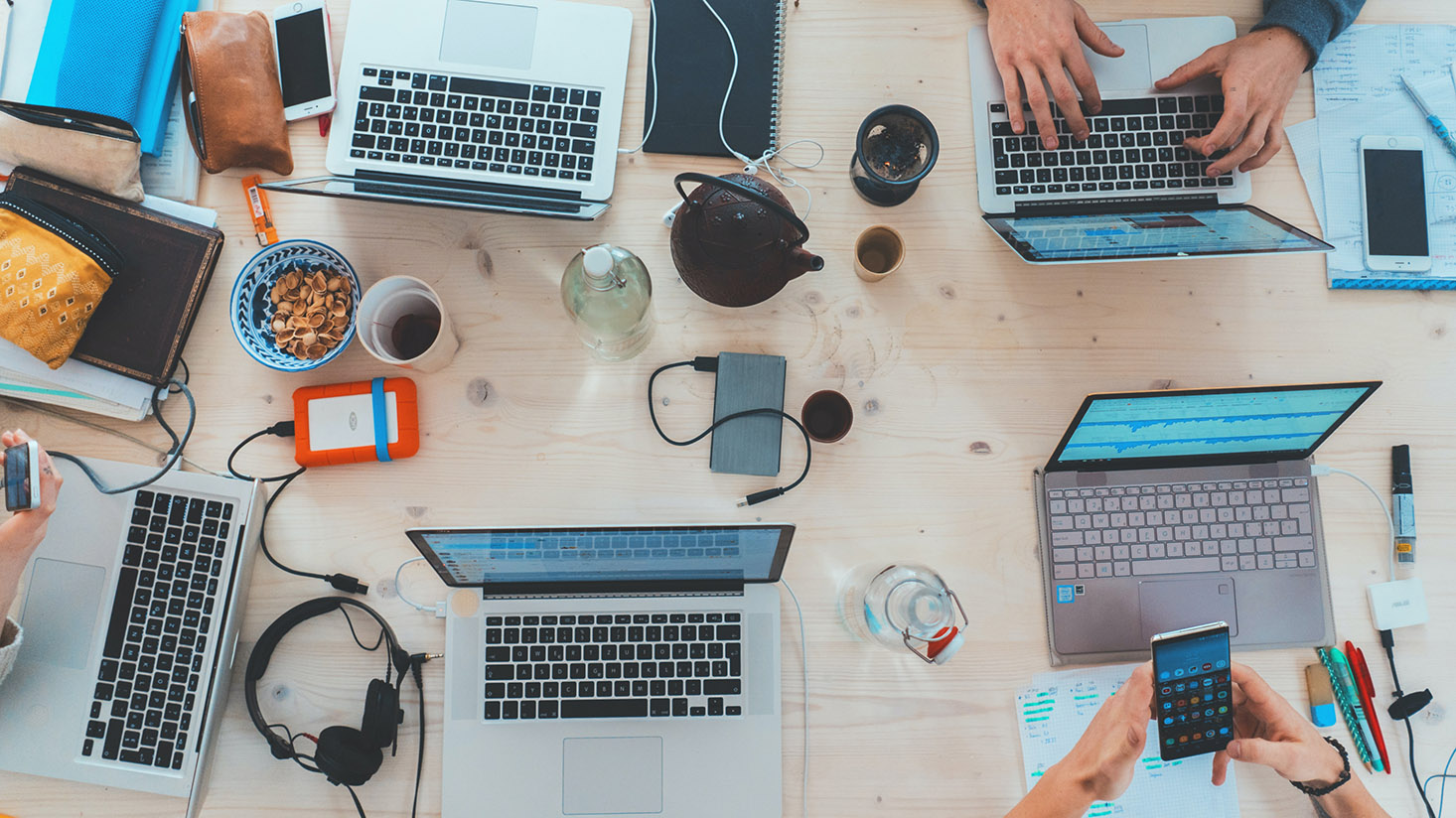
Introduction
Understanding customer behavior and predicting churn has always been a critical challenge for businesses, especially in sectors with high competition and customer turnover, such as telecommunications. Historically, companies relied on basic customer information and rudimentary analytics to gauge loyalty and predict churn. These methods often included manual surveys, feedback forms, and simple transaction records. Before the digital era, insights were primarily based on anecdotal evidence and limited customer interaction data, leading to reactive strategies rather than proactive churn prevention.
The advent of sensors, the internet, and connected devices has revolutionized data collection, enabling businesses to gather detailed insights about customer behavior, preferences, and dissatisfaction triggers. This shift towards digital data collection, coupled with the proliferation of software and database technologies, has allowed for the storage and analysis of vast amounts of event data, transforming how companies approach churn prediction.
Today, the importance of data in understanding and predicting customer churn cannot be overstated. Businesses are no longer in the dark, waiting weeks or months to understand changes in customer behavior. Real-time data analytics allow for immediate insights, enabling companies to address customer concerns proactively and tailor their offerings to increase customer retention.
Contact Data for Churn Prediction
The role of contact data in enhancing churn prediction models is pivotal. Historically, contact data was limited to basic information such as name, address, and phone number. However, with technological advancements, the scope of contact data has expanded significantly. Today, it encompasses a comprehensive 360-degree view of the customer, including both personal and business profiles.
Examples of modern contact data include:
- Personal and business profiles
- Demographic information
- Behavioral patterns
- Location data
Industries such as telecommunications have historically used contact data to manage customer relationships. However, the technology advances in data collection and analytics have transformed its use for churn prediction. The amount of contact data available is accelerating, providing deeper insights into customer behavior and preferences.
Specifically, contact data can be used to:
- Identify at-risk customers by analyzing changes in contact information, indicating potential life changes.
- Segment customers based on demographic and behavioral data for targeted retention strategies.
- Personalize communication and offers to increase customer engagement and loyalty.
Marketing Intelligence for Churn Prediction
Marketing intelligence data plays a crucial role in understanding customer behavior and predicting churn. This type of data provides insights into customer interactions with marketing campaigns, preferences, and engagement levels. Historically, marketing intelligence was gathered through customer surveys and market research. However, the digital transformation has enabled the collection of detailed marketing interaction data.
Examples of marketing intelligence data include:
- Customer engagement with marketing campaigns
- Product preferences and purchase history
- Customer feedback and satisfaction levels
Roles in marketing and customer relationship management have traditionally utilized marketing intelligence data to tailor campaigns and improve customer satisfaction. The advent of advanced analytics and machine learning has further enhanced the ability to predict churn based on marketing intelligence data.
Specific uses of marketing intelligence data in churn prediction include:
- Identifying patterns in customer engagement that may indicate dissatisfaction.
- Customizing retention campaigns based on customer preferences and feedback.
- Optimizing marketing strategies to improve overall customer experience and loyalty.
Conclusion
The importance of data in understanding and predicting customer churn has never been more evident. With access to diverse types of data, such as contact data and marketing intelligence, business professionals can gain deeper insights into customer behavior, preferences, and potential churn triggers. This wealth of information enables companies to develop targeted retention strategies, personalize customer interactions, and ultimately reduce churn rates.
As organizations become more data-driven, the ability to discover and leverage relevant data will be critical to success. The future of churn prediction lies in the integration of various data types, from demographic and behavioral data to real-time engagement metrics. Moreover, the potential to monetize valuable data assets opens new opportunities for businesses to enhance their churn prediction models and strategies.
Speculating on the future, the emergence of new data types and advanced analytics techniques, such as artificial intelligence (AI), will further revolutionize churn prediction. AI has the potential to unlock hidden insights in decades-old documents and modern digital interactions, providing unprecedented accuracy in predicting customer churn.
Appendix
Industries and roles that could benefit from enhanced churn prediction data include investors, consultants, insurance companies, market researchers, and more. These stakeholders face challenges in understanding customer behavior and predicting churn, which can significantly impact business outcomes. Data has transformed these industries by providing actionable insights that inform strategic decisions.
The future of these industries lies in the continued integration of data and AI technologies. By leveraging advanced analytics and machine learning, businesses can uncover patterns and trends that were previously undetectable, enabling more accurate and timely predictions of customer churn.