Neighborhood Spending Insights
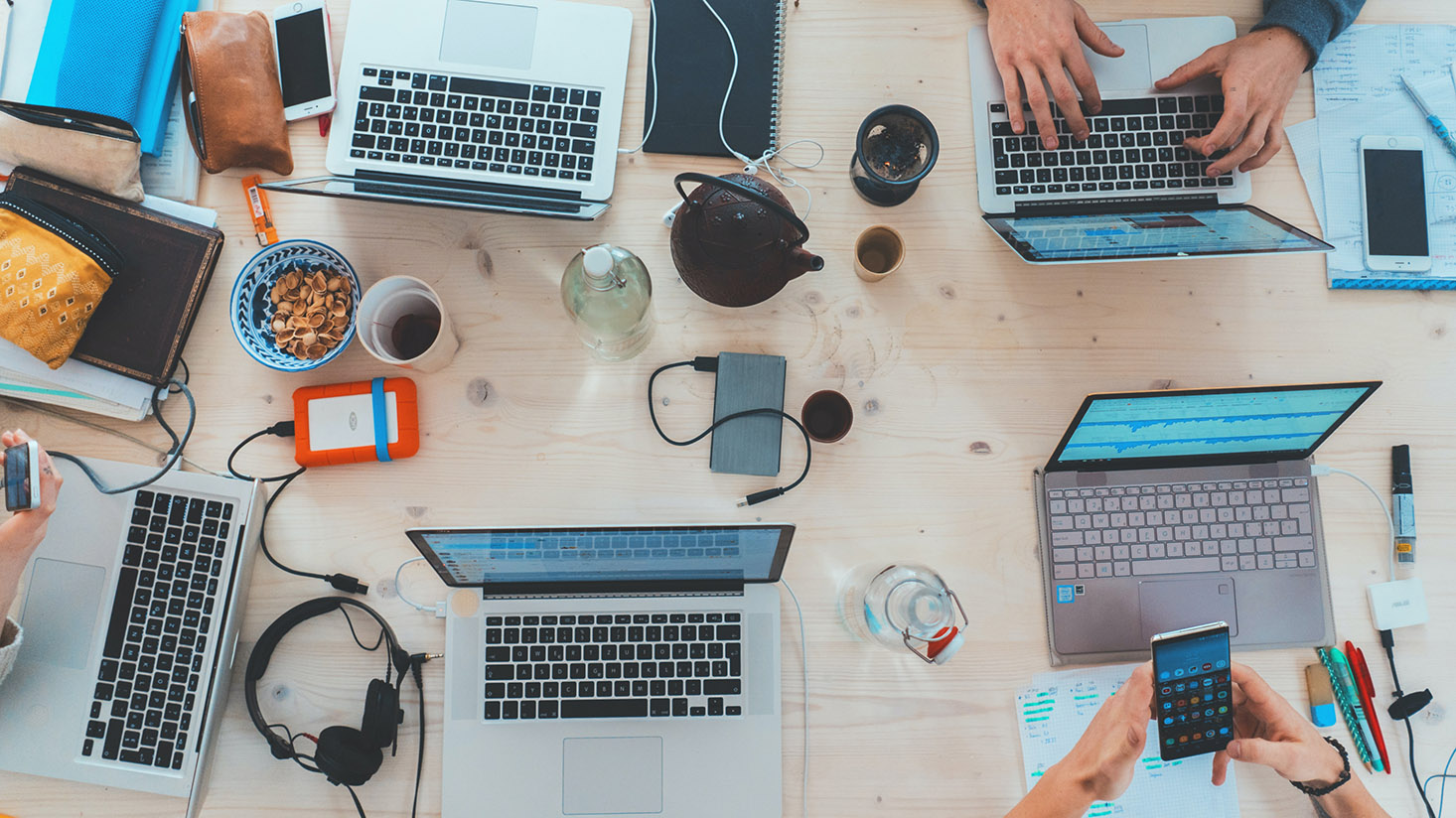
Introduction
Understanding consumer spending patterns at a granular level, such as by neighborhood or postal code, has historically been a challenging endeavor. Before the digital age, businesses and analysts relied on rudimentary methods to gauge market trends and consumer behavior. These methods ranged from conducting surveys and manual tallying of sales at local stores to making educated guesses based on observable economic indicators. The lack of precise data meant that businesses were often in the dark, making decisions based on outdated or incomplete information, leading to weeks or even months of delay before understanding shifts in consumer behavior.
The advent of sensors, the internet, and connected devices, alongside the proliferation of software into many processes, has revolutionized the way we collect and analyze data. This digital transformation has enabled the storage of every little event happening in some type of database, providing a wealth of data that was previously unimaginable. The importance of data in understanding consumer spending patterns cannot be overstated. It has illuminated previously dark corners of consumer behavior, allowing businesses to understand changes in real-time and make informed decisions swiftly.
Historically, without data, businesses relied on anecdotal evidence and slow, labor-intensive research methods to understand market trends. The introduction of connected devices and the internet has made it easier to collect and analyze data, providing insights that were previously out of reach. This shift towards data-driven decision-making has been transformative, enabling businesses to react quickly to changes in consumer behavior and market trends.
The importance of data in gaining insights into neighborhood spending patterns is clear. It allows businesses to understand where people are spending their money, how much they are spending, and on what they are spending it, without needing the names of individual merchants. This level of detail, aggregated at the business category level, provides a clear picture of consumer behavior within specific neighborhoods, enabling businesses to tailor their strategies accordingly.
Before the availability of such data, businesses had to rely on less precise methods, such as customer surveys or sales data from a limited number of sources. These methods provided a fragmented view of consumer spending, often leading to inaccurate or incomplete insights. The digital revolution has changed this, providing access to comprehensive and up-to-date data that offers a complete picture of consumer spending patterns.
The transition from antiquated methods to modern, data-driven approaches has been a game-changer for businesses seeking to understand neighborhood spending patterns. The ability to analyze data in real-time has provided businesses with the insights needed to make informed decisions, adapt to changing market trends, and stay ahead of the competition.
Transaction Data
History and Evolution
Transaction data has been a critical component in understanding consumer spending patterns. Historically, this data was collected through manual sales logs and receipts, providing a fragmented and often delayed view of consumer behavior. The technology advances in point-of-sale systems, online transactions, and data analytics have revolutionized the collection and analysis of transaction data. The amount of data available has accelerated, offering businesses unprecedented insights into consumer spending.
Examples of Transaction Data:
- Point-of-Sale Transactions: Data collected from physical and online sales, providing insights into what consumers are buying.
- Credit Card Transactions: Aggregated and anonymized data showing spending patterns across different business categories.
- Online Purchases: Data from e-commerce platforms, offering insights into online shopping behavior.
Roles and Industries: Retailers, e-commerce platforms, financial institutions, and market researchers have historically used transaction data to understand consumer behavior, tailor marketing strategies, and improve product offerings.
Technology advances, such as the development of sophisticated data analytics tools and the widespread adoption of digital payment methods, have made transaction data more accessible and valuable. The ability to analyze this data in real-time has provided businesses with the insights needed to respond quickly to changing consumer preferences and market trends.
Using Transaction Data for Neighborhood Spending Insights:
- Benchmarking: Comparing spending patterns across different neighborhoods to identify trends and opportunities.
- Category Performance: Analyzing spending in specific business categories, such as grocery or restaurants, to understand consumer preferences.
- Competitive Analysis: Understanding how businesses in the same category perform relative to each other within a neighborhood.
- Location Performance: Identifying high-performing and underperforming areas based on consumer spending patterns.
Diversified Data
History and Evolution
Diversified data encompasses a wide range of data types, including consumer panels, social media activity, and location data. This category of data has grown significantly with the advent of the internet and mobile devices, providing a more comprehensive view of consumer behavior. The ability to aggregate and analyze data from various sources has offered businesses deeper insights into consumer spending patterns, preferences, and trends.
Examples of Diversified Data:
- Consumer Panels: Data collected from groups of consumers who provide insights into their purchasing behavior over time.
- Social Media Activity: Insights into consumer preferences and trends based on social media interactions and discussions.
- Location Data: Information on consumer movements and behaviors based on mobile device data.
Roles and Industries: Marketing agencies, retail businesses, and consumer goods companies use diversified data to gain a deeper understanding of consumer behavior, enhance customer engagement, and drive sales.
The proliferation of digital devices and platforms has made diversified data more accessible, providing businesses with a holistic view of consumer behavior. The integration of data from various sources has enabled more accurate and timely insights, allowing businesses to make data-driven decisions and stay competitive in a rapidly changing market.
Using Diversified Data for Neighborhood Spending Insights:
- Understanding Consumer Preferences: Analyzing data from consumer panels and social media to identify trends and preferences within specific neighborhoods.
- Enhancing Customer Engagement: Leveraging location data and consumer insights to tailor marketing strategies and improve customer experiences.
- Driving Sales: Identifying opportunities for growth based on comprehensive analysis of consumer behavior and spending patterns.
Conclusion
The importance of data in understanding neighborhood spending patterns cannot be overstated. The transition from antiquated methods to modern, data-driven approaches has provided businesses with the insights needed to make informed decisions, adapt to changing market trends, and stay ahead of the competition. The availability of transaction data and diversified data has revolutionized the way businesses understand consumer behavior, offering a level of detail and accuracy that was previously unattainable.
As organizations become more data-driven, the ability to discover and analyze relevant data will be critical to their success. The trend towards monetizing useful data that businesses have been creating for decades is on the rise, and neighborhood spending patterns are no exception. The future will likely see the emergence of new types of data that can provide additional insights into consumer behavior, further enhancing the ability of businesses to make data-driven decisions.
The role of data in understanding neighborhood spending patterns is clear. It provides businesses with the insights needed to tailor their strategies, improve customer engagement, and drive sales. The continued evolution of data analytics and the increasing availability of diverse data sources will undoubtedly play a crucial role in shaping the future of business strategy and consumer understanding.
Appendix
Industries and roles that could benefit from data on neighborhood spending patterns include investors, consultants, insurance companies, market researchers, and more. These professionals face challenges in understanding market trends and consumer behavior, and data has transformed the way they approach these challenges.
The future holds great potential for unlocking the value hidden in decades-old documents or modern government filings through AI and advanced analytics. As businesses and industries continue to evolve, the importance of data in driving decision-making and strategy will only increase, highlighting the critical role of data in understanding and responding to consumer behavior and market trends.