Training Data for Entity Recognition
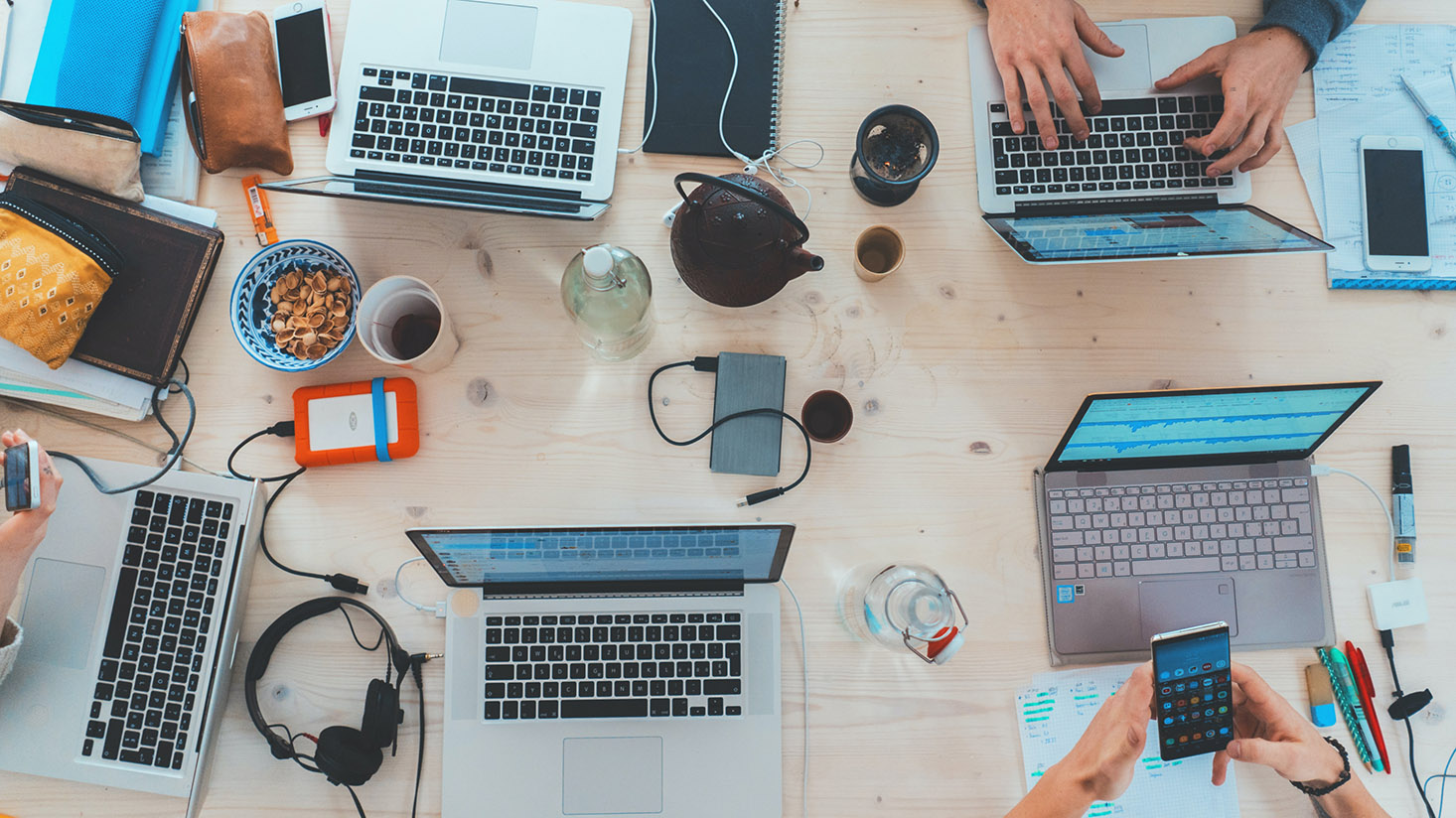
Introduction
In the realm of artificial intelligence and machine learning, the quality and specificity of training data can significantly impact the performance of models, especially in tasks such as entity recognition. Historically, acquiring high-quality, domain-specific datasets for training purposes was a formidable challenge. Before the digital era, data collection was labor-intensive and often relied on manual methods, such as transcribing conversations, manually annotating texts, or compiling records from physical documents. The advent of the internet, sensors, and connected devices, alongside the proliferation of software across various industries, has revolutionized data collection and storage. This digital transformation has enabled the accumulation of vast amounts of data, making it easier to obtain specific datasets for training AI models.
Previously, professionals and researchers were in the dark, waiting weeks or months to gather and analyze data to understand patterns or changes in their fields of interest. Now, with real-time data collection and processing, insights can be gleaned almost instantaneously. This shift has been particularly transformative in fields requiring nuanced understanding of text data, such as customer support, healthcare, and legal industries. The ability to train models on large corpora of text data allows for more accurate and efficient entity recognition, which is crucial for tasks ranging from customer service automation to patient data management and legal document analysis.
AI Training Data Provider
The emergence of AI Training Data Providers has been a game-changer for developing entity recognition models. These providers specialize in compiling and organizing large datasets tailored to specific needs, such as customer support calls, medical notes, and legal contracts. The technology advancements in data anonymization and tagging have made it possible to use sensitive information responsibly, ensuring privacy while still providing valuable training material.
- History and Evolution: Initially, training data was scarce and often not specific enough for nuanced tasks. The development of specialized data providers has enabled the collection of vast, domain-specific datasets.
- Examples of Data: This includes transcriptions of customer support calls, deidentified medical notes, and legal contracts, all of which can be pre-tagged to facilitate model training.
- Industry Usage: Industries such as healthcare, legal, and customer service have historically benefited from these datasets, using them to train models for tasks like automated customer support, patient data analysis, and contract review.
- Technology Advances: Advances in natural language processing (NLP), data anonymization, and tagging technologies have been crucial in the development and utilization of these datasets.
- Accelerating Data Volume: The volume of available training data is accelerating, thanks to digital transformation across industries and the increasing capabilities of data providers to compile and organize large datasets.
- Usage for Entity Recognition: These datasets are instrumental in training models for entity recognition, enabling more accurate identification of specific entities within texts across various domains.
Healthcare Data Provider
Healthcare Data Providers offer a wealth of deidentified clinical notes and reports, which are invaluable for training models in medical entity recognition. The availability of such detailed and specific datasets allows for the development of models capable of understanding and processing complex medical terminology and patient information.
- History and Evolution: The transition from paper-based to digital medical records has significantly increased the availability of healthcare data for training purposes.
- Examples of Data: Datasets like MIMIC-IV-Note, containing millions of deidentified clinical notes and radiology reports, are examples of the rich data available for model training.
- Industry Usage: These datasets are particularly useful for healthcare professionals and researchers working on NLP projects aimed at improving patient care and medical research.
- Technology Advances: Advances in data deidentification and NLP have made it possible to use sensitive healthcare data responsibly for training purposes.
- Accelerating Data Volume: The digitization of healthcare records continues to accelerate, providing an ever-growing corpus of data for training AI models.
- Usage for Entity Recognition: Training models on these datasets enables more accurate recognition of medical entities, facilitating tasks such as automated patient data analysis and diagnosis assistance.
Conclusion
The importance of high-quality, domain-specific training data in developing effective entity recognition models cannot be overstated. As industries continue to digitize and generate vast amounts of data, the role of specialized data providers in compiling, organizing, and anonymizing this data becomes increasingly crucial. Access to such datasets allows business professionals and researchers to train more accurate and efficient models, ultimately leading to better decision-making and improved outcomes in various fields.
Organizations are becoming more data-driven, recognizing the value of data not only for internal purposes but also as a potential revenue source. As this trend continues, we can expect to see an expansion in the types of data available for training purposes, further enhancing the capabilities of AI models in entity recognition and beyond. The future of data-driven insights is bright, with advancements in AI and machine learning poised to unlock the value hidden in decades-old documents and modern digital records alike.
Appendix
Industries and roles that stand to benefit from access to high-quality training data include investors, consultants, insurance companies, market researchers, and more. These stakeholders face various challenges that can be addressed through better data-driven insights. For example, investors can use entity recognition models to analyze market trends, while consultants may leverage them for competitive analysis.
The future of these industries lies in the integration of AI and machine learning technologies, which can unearth valuable insights from vast datasets. As the volume and variety of data continue to grow, the potential for AI to transform these fields is immense, promising a new era of innovation and efficiency.