Unlocking Insights into Sugar Pricing with Diverse Data Sources
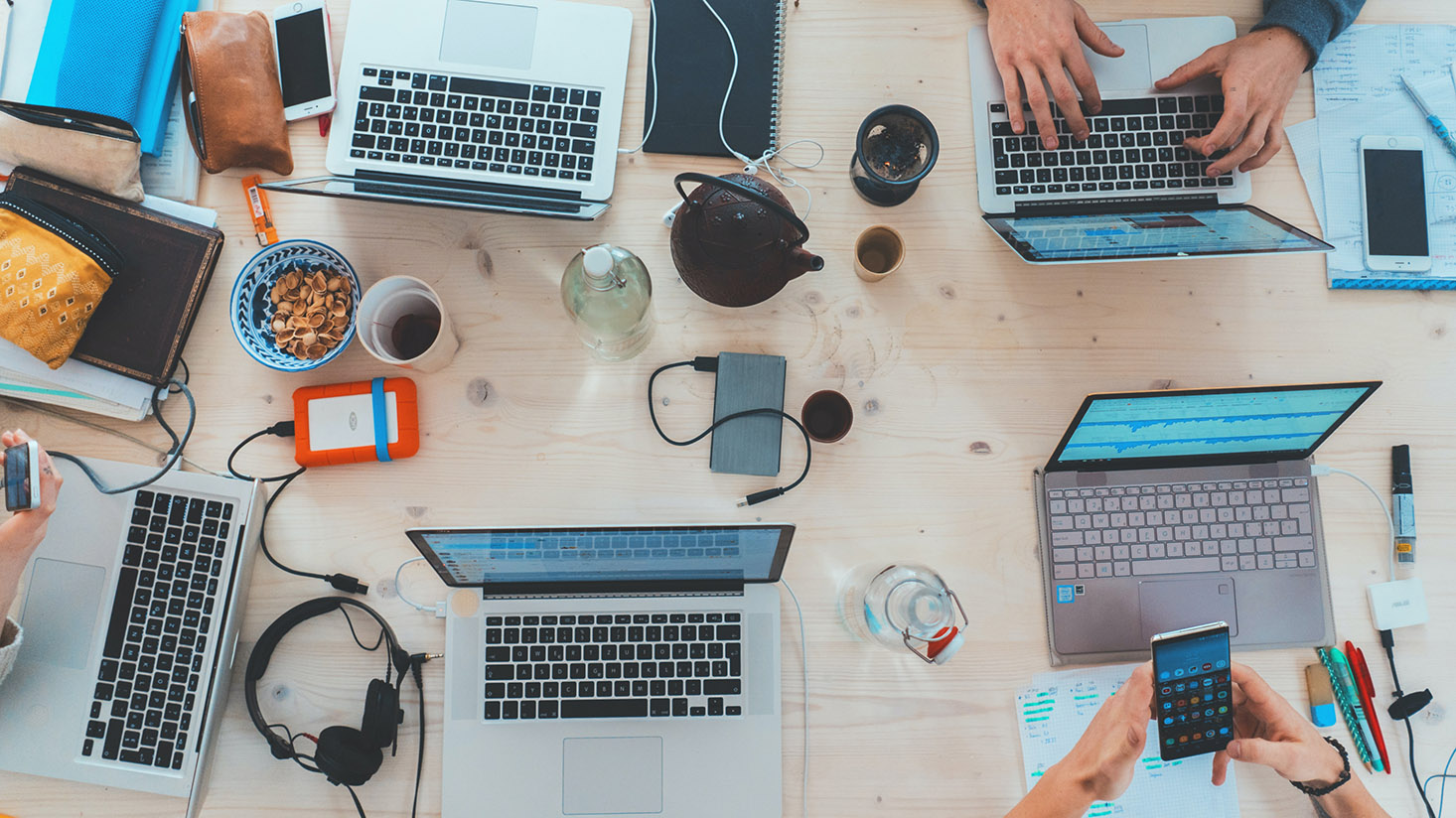
Unlocking Insights into Sugar Pricing with Diverse Data Sources
Introduction
Understanding the intricate web of sugar pricing, particularly within the United States, has long been a complex puzzle for businesses and analysts alike. Historically, the opaque nature of sugar pricing, especially non-futures wholesale prices, left businesses in a fog when attempting to make informed decisions. In the days before the advent of expansive modern data and sensors, sugar pricing insights were gleaned through word of mouth, sporadic governmental reports, and outdated commodity exchanges.
Before the technological revolution, firms relied on anecdotal evidence, often depending on agricultural studies or fragmented local market reports. The challenge was evident; without a standardized data source, businesses had to be patient—or rather stagnant—while awaiting sporadic updates or unofficial estimates. Evaluating price trends or market fluctuations felt like searching for a needle in a haystack.
The emergence of the internet and connected devices revolutionized the potential for real-time data acquisition. Suddenly, price variations and market trends could be tracked instantaneously through sophisticated external data analytics platforms. What had once taken weeks or months to discern—if it was discernable at all—could now be illuminated in real-time, offering businesses an edge they hadn't previously imagined.
This transformation was catalyzed not just by the internet, but by the suite of technologies that followed. Sensors, distributed networks, and digital databases proliferated, creating a treasure trove of actionable data. The availability of real-time data radically reshaped the landscape, offering a 360-degree view of sugar price dynamics.
Data's role has become indispensable in deciphering sugar pricing within the U.S. Thanks to the evolution of data types—from financial and commodities to satellite and environmental—professionals can now dissect price mechanisms with remarkable clarity. No longer are they working in the dark; they possess the clarity to act swiftly and strategically.
Today, the intersection of various categories of data has filled the information void that once left professionals guessing. These data sources not only afford a comprehensive understanding of sugar pricing but also empower businesses to leverage insights for competitive advantage.
Financial Data
Financial data has been a cornerstone of market analysis for decades. Traditionally, it revolved around stock exchanges and market indices, which provided a lagging view of economic conditions. Historical sugar price assessments required familiarity with futures exchanges—chiefly used for global rates, not the specific U.S. market prices under discussion today.
With technological advances, financial data has evolved to become more granular and near-real-time. Extensive datasets now capture market transactions, contract assessments, and comprehensive pricing models. This evolution ushered in unprecedented clarity and consistency in how firms could monitor economic trends.
Leading examples of financial data leverage algorithms and historical datasets to benchmark sugar pricing. Such data has widespread applications across industries—from food manufacturers to financial institutions—providing them with the necessary leverage to predict and respond to market shifts.
The march toward more comprehensive financial data was accelerated with the integration of market intelligence platforms. These platforms now afford access to real-time financial analytics tools, offering insights that drive critical decision-making.
Specifically, financial data provides the following insights into the sugar prices:
- Market Trends: Financial datasets highlight trends and anomalies in sugar pricing distinct from global futures rates.
- Trading Strategies: Businesses can harness these insights to optimize trading strategies and mitigate market risks.
- Risk Management: Through benchmark data, firms are equipped to strategize and reduce basis risk.
- Granular Analysis: Historical data supports backtesting and strategic forecasting.
- Asset Optimization: Financial insights inform treasury and asset optimization, impacting planning and resource allocation.
Commodities Data
The commoditization of agricultural data transcended traditional bounds once restricted by physical and logistical limitations. Historical commodity data focused on global indices, often overlooking localized specifics crucial for enterprises operating within the U.S.
Commodities data underwent a renaissance with advancements in global communication networks and data collection technologies. Platforms now aggregate comprehensive data that spans production, trade, seasonality, and more, thus capturing a holistic view of the agricultural market.
These datasets, when carefully curated and analyzed, illuminate critical pricing patterns and seasonal shifts. Agricultural firms and analysts extensively use this data to inform production schedules, market entry strategies, and pricing paradigms.
With an ever-growing demand for precision, commodities data platforms have continually expanded the scope and accuracy of the information they provide. From ephemeral market prices to enduring historical data, they have become a staple in analyzing agriculture.
For instance, commodities data can provide insights into sugar pricing in several impactful ways:
- Domestic Pricing Analysis: This data offers a comprehensive view of U.S. wholesale sugar prices—crucial for tracking cost fluctuations.
- Seasonality Patterns: Understanding seasonal trends helps businesses adjust buying and supply chain strategies.
- Production Influences: Analyzing production data correlates environmental or economic factors to pricing variations.
- Trade Dynamics: Global trade patterns influence domestic scenarios, offering a broader context to sugar pricing.
- Localized Intelligence: Region-specific data identifies unique pricing factors affecting sugar costs within the U.S.
Conclusion
The role of data in understanding sugar pricing within the U.S. measures far beyond mere commodity tracking. With complex integrations of financial and commodities data, businesses can now harness a multidimensional view of pricing dynamics to better strategize and optimize operations.
Access to diverse data types enables businesses to make more informed decisions, an essential capability in today's fast-paced environment. This approach not only enriches their strategic toolbelt but also propels them into the future, where decisions are data-driven, and successes are scalable.
The proliferation of data monetization efforts further emphasizes the value firms place on actionable insights. Many data providers are looking to monetize their data, reflecting this emerging capitalization trend and the expanding influence data had and continues to have.
As more businesses wake up to the value of AI and advanced analytics, organizations are increasingly vitalizing corporate strategies around data discovery. These transformations are being harnessed to remain competitive and resilient within constantly shifting market landscapes.
Indisputably, the future is bright for businesses capitalizing on AI and data synergies. Innovation will spark further data types from companies keen on maximizing the insights into market prices, be it sugar or another valuable commodity.
Appendix
Many industries and roles stand to gain from the nuanced understanding of sugar pricing data available today. Investors, for instance, benefit from accurate wholesale price predictions to optimize their portfolios and mitigate risks associated with market volatility.
Consultants and market researchers work closely with businesses in food and beverage, aiding them with strategic insights and industry forecasts that inform product development and pricing strategies. These professionals harness external data to offer data-driven recommendations that strengthen competitive advantage.
Insurance companies also find immense value in sugar pricing data. Their risk assessment models are enhanced by accurately tracking agricultural commodities, providing insurance products tailored to client needs within the fragility of volatile markets.
The entire supply chain from producers to retailers benefits from insights into sugar pricing and trends. Such detailed pricing data informs every phase of logistics management, from crop output through distribution to final product placement.
The future potential of data analytics and AI could unlock insights from historical documents, presenting a monumental opportunity for data-driven decision-making. As firms digitize data that has been dormant for years and the government releases new filings, the potential for discovery is immense.
Overall, the synergy of various data types, analytics, and the growing role of artificial intelligence remains a fundamental driver of industry progress and adaptation. The enterprise-wide embrace of data as a central asset underscores the timeless relevance of reliable insight in navigating complex economic landscapes.